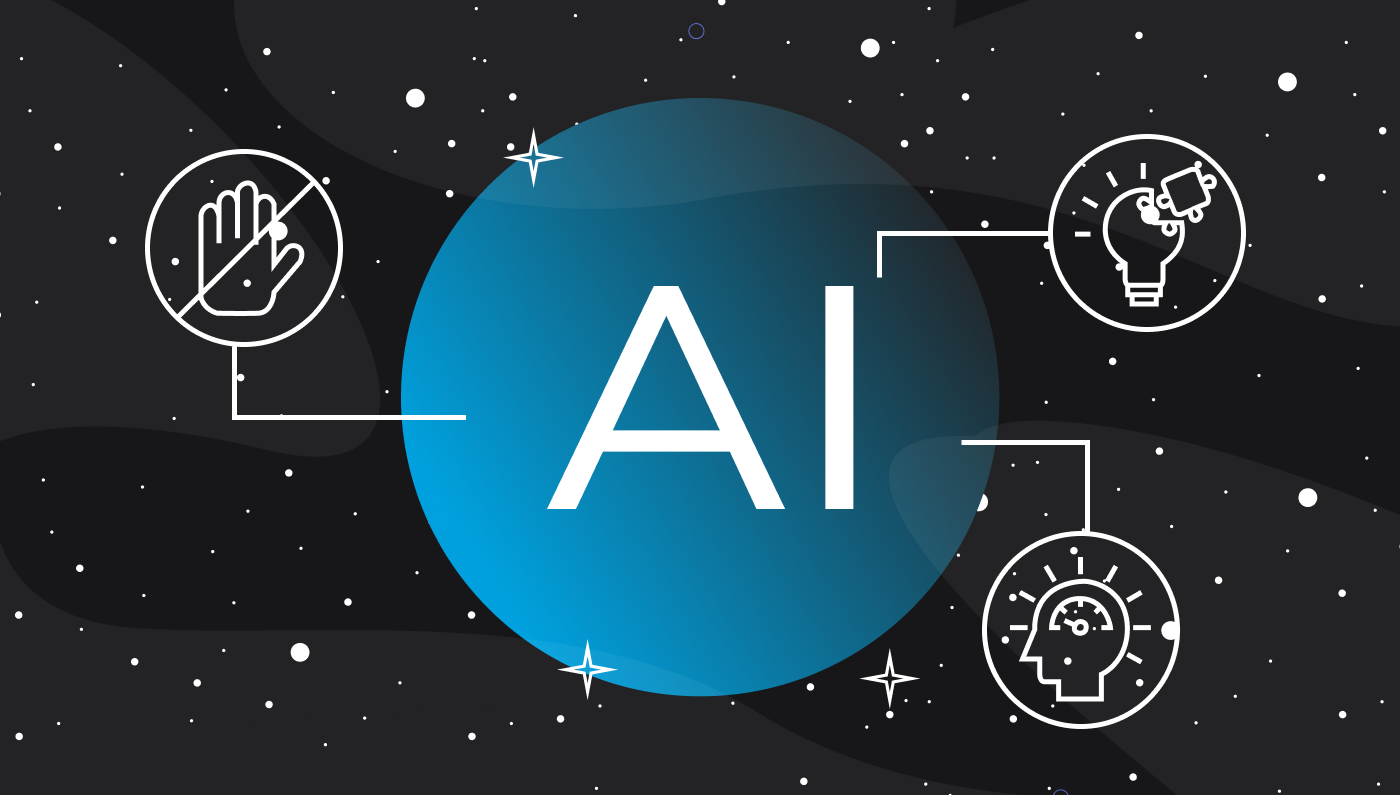
The Challenges, Limitations and Potential of AI Trading
The financial services sector has been one of the most enthusiastic adopters of artificial intelligence (AI) technology. Machine-learning, a subset of AI, has been a critical tool in providing a competitive advantage for algorithmic traders with its ability to process and analyze massive volumes of data incredibly quickly. It has also been a valuable tool for financial institutions in combating money-laundering and fighting financial crime. However, there are challenges that still must be overcome, so caution is advised when using AI to manage or facilitate your trading.
AI Trading Limitations
Machine learning algorithms can now manage every aspect of your trading for you, learning and improving along the way. They have the capacity to decide where to buy and sell, as well as what to buy and sell. They can analyze huge quantities of financial data, identify opportunities, and autonomously execute orders by accessing multiple liquidity sources at once. This is incredibly exciting and, potentially, opens the door to low-risk investment for those with some capital to invest but little financial experience to guide them.
However, placing all your trust and hard-earned capital in the hands of a machine clearly entails risks. Could you lose everything, as well as money you don’t have, on bad trades? What about a technical glitch? What if the AI trading bot is trained on flawed, low-quality datasets?
Many AI experts suggest the utilization of supervised machine learning, which places narrow guardrails on the coded activities a machine can perform, so it isn’t processing and interpreting data alone. This could include limits on the data sources they are allowed to analyze, on the types of trading they are allowed to perform, and on the amount of trading capital they are allowed to invest per trade or per day.
The Regulatory Challenges of AI Trading
While the energence of AI has brought faster transactions and therefore increased market liquidity, it has also created a number of regulatory challenges for the financial sector.
The Complexity of AI Oversight
One major challenge is the complexity of supervision when it comes to machine learning algorithms. For example, as machine learning algorithms evolve, they become increasingly hard to audit, and their behavior is more difficult to predict, potentially leading to higher volatility and greater trading risk.
Adaptability to Unfamiliar Conditions
Another issue is whether an AI that is trained in specific market conditions can trade effectively under conditions it has not yet learned to navigate. For example, can a machine learning algorithm trained in a period of relative market stability perform equally well if the market suddenly enters a highly volatile period? Will it be able to anticipate the undervaluation of stocks in an economy with rising inflation if it has only been trained in an era of low inflation?
The Dangers of Uncontrolled Learning
While the Hollywood movie scenario of machines evolving beyond our control and enslaving humanity is rather unlikely, there is a real risk of uncontrolled learning. This is a situation in which algorithms leave humans behind to the point where developers are unable to fully comprehend their decision-making processes.
The solution is to effectively determine which tasks should be left to a bot and which should still be performed by humans. For example, creating long-term investment strategies and building a portfolio safely should involve some degree of oversight.
Source Data Quality and Quantity
Probably the main risk in maintaining an algorithmic trading bot’s trade success rates is ensuring that there is enough quality source data in substantial quantities. The more reliable, relevant data from diverse sources a machine learning algorithm is fed, the better. Also, the longer its history using the data to formulate strategies, execute trades, and learn from each outcome, the more precisely it can predict market patterns and anticipate price shifts.
On the upside, particularly in the world of AI trading, there is a wealth of available source data. Also, AI-based bots can operate with only a few months of historic data.
However, just like people, algorithmic trading systems rely on what they learned in the past to make decisions about the future. Put simply, their decision-making skills are only as good as the history on which they are based. Moreover, if previously unseen trading conditions arise, the bot may be ill-equipped to respond. The best way to avoid this pitfall would be to fit the data to longer periods.
AI Trading’s Potential
It would be almost impossible to argue that the risks of AI trading outweigh the rewards. The primary benefit of AI is its ability to absorb and analyze a wealth of data at an inhuman speed, which can have a huge impact in areas like trade pricing, order execution, sentiment analysis, portfolio management, and high-frequency trading.
Machine learning is perfectly suited to respond in real time to price shifts in low-latency systems. Traders at every level are taking advantage of AI’s unique capacity to detect patterns and anomalies across multiple markets simultaneously when it is given large volumes of quality data.
As a result of these advantages, banks looking to detect fraud and investment firms aiming to optimize revenue while minimizing risk share the increasingly popular view that if it can be automated, then automate it!
Here at AiStakes, our automated algorithmic trading bot uses machine learning and natural language processing (NLP) models to trade on the user’s behalf. The algorithmic trading bot is trained on massive datasets from a wide range of traditional and alternative data sources, including global macro news and country, currency, and commodity-specific data. It learns as it goes, minimizing risk and optimizing profits more effectively with each trade, so it now has a win/loss ratio exceeding 80%.
Registered in the EU and authorized to provide financial services, including crypto, AiStakes diversifies its trading across forex, stocks, commodities, indices, and bonds. Our algorithmic trading app uses a wealth of risk management measures, from stops and limits and automated hedging tools to caps on the amount allocated per trade and a reserve fund providing client capital coverage and partial compensation on losing trades.
In addition, there is human oversight over the algorithm, with adjustments being made when necessary, particularly in periods of high volatility or in a rising inflation marketplace. It is constantly being improved, and the mathematical models are refined to suit evolving market conditions.
To find out more about AI, algorithmic trading, market analysis, blockchain, and crypto, as well as a wide array of traditional asset classes, check out the AiStakes blog.