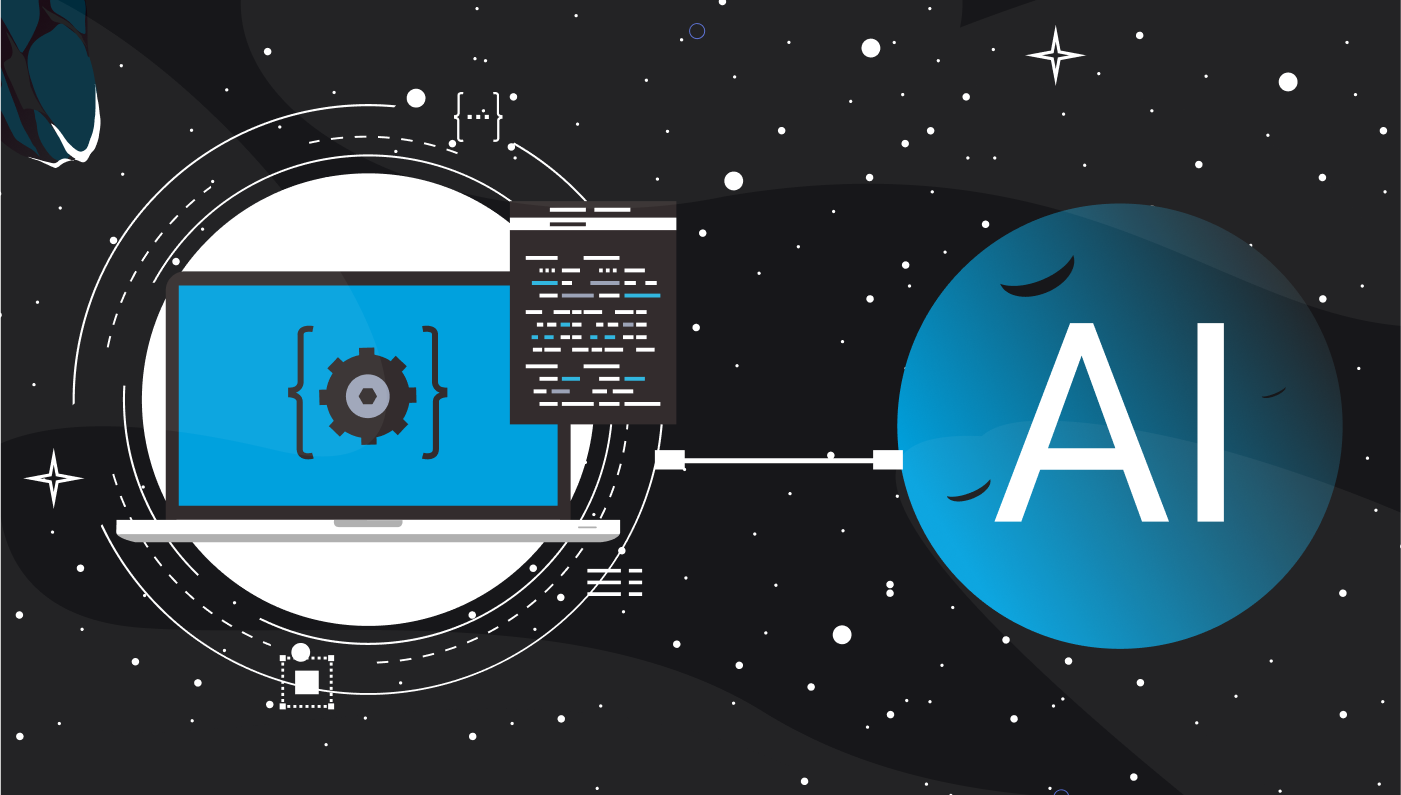
AI Quantitative Trading Explained
There has been a great deal of buzz recently surrounding AI quantitative trading so before we dig in to how it works, its future potential, and whether it can live up to the hype, let’s define what it is.
What Is AI Quantitative Trading?
Quantitative trading, or quant trading for short, is a means of creating trading strategies based on complex mathematical computations. It involves quantitative analysis, which identifies market opportunities using mathematical and statistical modeling, measurement, and research to understand and anticipate market behavior. Quant traders will input multiple data points, such as historic price and volume data into mathematical models for the creation of trading algorithms.
A hot topic in quant trading these days is Artificial Intelligence (AI). AI is an evolving field that concerns itself with the simulation of human thought processes by machines, and in particular computer systems. A specific subset of AI is Machine Learning (ML), which relates to the ability of a machine to learn from data and adapt to new scenarios, without explicit programming.
Both trading and machine learning are rooted in mathematics and are statistically driven, making them a natural match.
How Does AI Trading Work?
When AI is combined with quantitative trading algorithms, the result is a trading bot that can increasingly successfully anticipate market patterns and trends. It makes trading decisions by processing a vast amount of market-related data, constantly learning from its own trading activity and from the market information it receives.
As AI continues its rapid evolution, computer processing power advances, and big data takes off, the predictive power of AI quantitative trading is going from strength to strength. A bot is only as good as the data it is fed, and high-quality big data sets will mean more scalability and a far greater predictive accuracy.
The use of AI in quantitative trading, and particularly developments in machine learning, have changed the game for traders, improving almost every aspect of the process from market forecasting, data processing, and modeling, to risk analysis, signals research, and trade execution.
The sheer quantity of data being generated globally is also growing by hundreds of percent each year. Specifically, the markets are seeing a growth in alternative sources of data, now used by approximately 70% of hedge funds, which is information collected from nontraditional sources, such as satellite imagery, credit card transactions, product reviews, and social media commentary.
The incredible processing capabilities of AI quantitative trading enable bots to crunch vast amounts of information at lightning speed, making it increasingly valuable in a data driven world. The way it works is that alternative data is used in conjunction with machine learning to find new AI trading signals or improve those that already exist.
Examples of AI trading signals being created from alternative data include the use of credit card information in stocks trading, satellite images of farming land in commodities trading and even, as in one particularly famous instance, using air traffic data to predict mergers and acquisitions. New potential factors impacting the market are still being uncovered all the time, and AI has proven to be a leader in this journey of discovery. In addition, Machine Learning can combine non-linear signals or pool together multiple weak predictors. However, issues of privacy and data rights may lead to stricter regulation in this area, moving forward.
What Does the Future Look Like for AI Trading?
One of the biggest challenges facing AI trading is the complexity, scale, and dynamic nature of the financial markets. Even with the already huge processing modelling and forecasting potential of AI quantitative trading technology, there is still a long way to go before artificial intelligence is able to flawlessly predict trends to guarantee market success.
One large stride forward has come with the introduction of generative AI language models like ChatGPT, that use Natural Language Processing (NLP) and deep learning capabilities. So, based on the data they are fed, they can learn by example and then make inferences independently as new data is input. These models can be trained on financial news, price and volume data, social media posts that can give insight into market sentiment and more, to generate predictions about asset price movements.
Here at AiStakes we have incorporated generative AI models, with our proprietary algorithms to enable real-time analysis and decision-making. Trained on a wide variety of structured and unstructured, alternative market-related data sources, our bot, ALGOS can increasingly accurately predict market trends and make smart, informed market decisions on our clients’ behalf.
To learn more about a wide array of topics relating to AI, financial assets and the world of trading, check out the AiStakes blog.